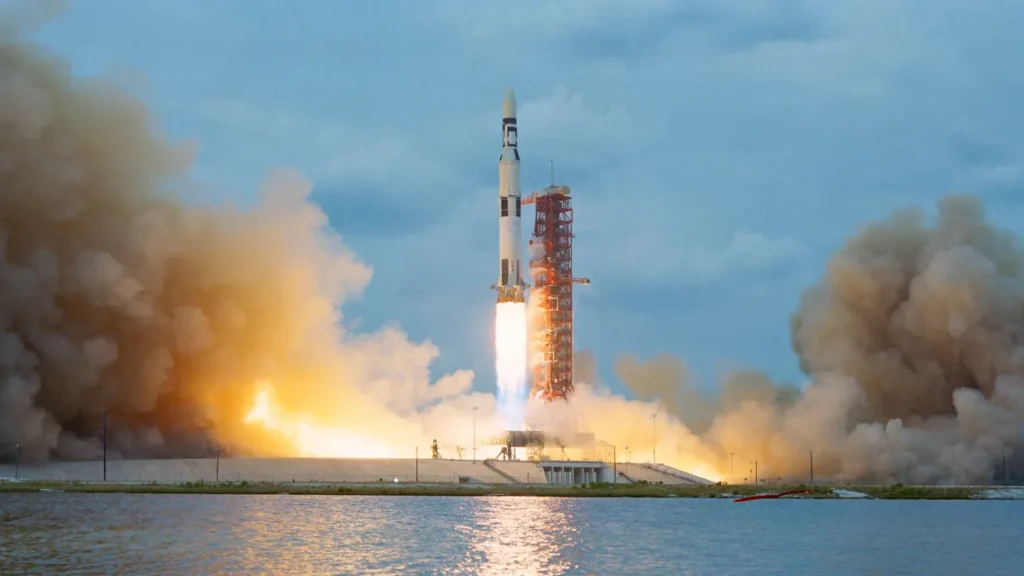
Executive Overview
As global markets evolve at breakneck speed, Fortune 500 companies face a crucial imperative: to harness the transformative power of AI as a core strategic asset. AI is no longer merely an efficiency tool—it is reshaping business models, driving innovation, and redefining competitive advantage. This article offers a comprehensive, multi-dimensional framework for integrating AI into every facet of an enterprise’s growth strategy. By examining the underlying data, organizational shifts, and ecosystem recalibrations required for true AI integration, we reveal how companies can unlock sustainable growth, mitigate risks, and build an AI-native culture that delivers measurable results over time.
1. Reassessing the Foundations of Growth in an AI-Inflected Economy
1.1 The Transformation Imperative
Before AI, growth strategy has centered on incremental innovation, market expansion, and cost reduction. Yet the advent of advanced AI models—from deep learning to generative AI—is triggering a paradigm shift. In this new era, the focus moves from simply scaling operations to reinventing entire value networks. Enterprises like Hitachi illustrate this transformation. Once a traditional hardware manufacturer nearing bankruptcy in 2009, Hitachi has reinvented itself by monetizing data and deploying AI-enabled platforms such as Lumada, which have propelled its market value to over $100 billion in just two years (Hitachi Transformation)
This metamorphosis is not isolated. Other global players are pivoting similarly: Yum Brands is leveraging its Byte by Yum! platform to reimagine customer experience and operational efficiency (Yum Brands AI), while Dell has doubled down on AI infrastructure investments to drive a 34% revenue jump in its Infrastructure Solutions Group (Dell AI & RTO). These examples underscore that AI’s true value lies in its ability to rewire the very DNA of business growth strategy—from the frontline customer experience to back-end operational processes.
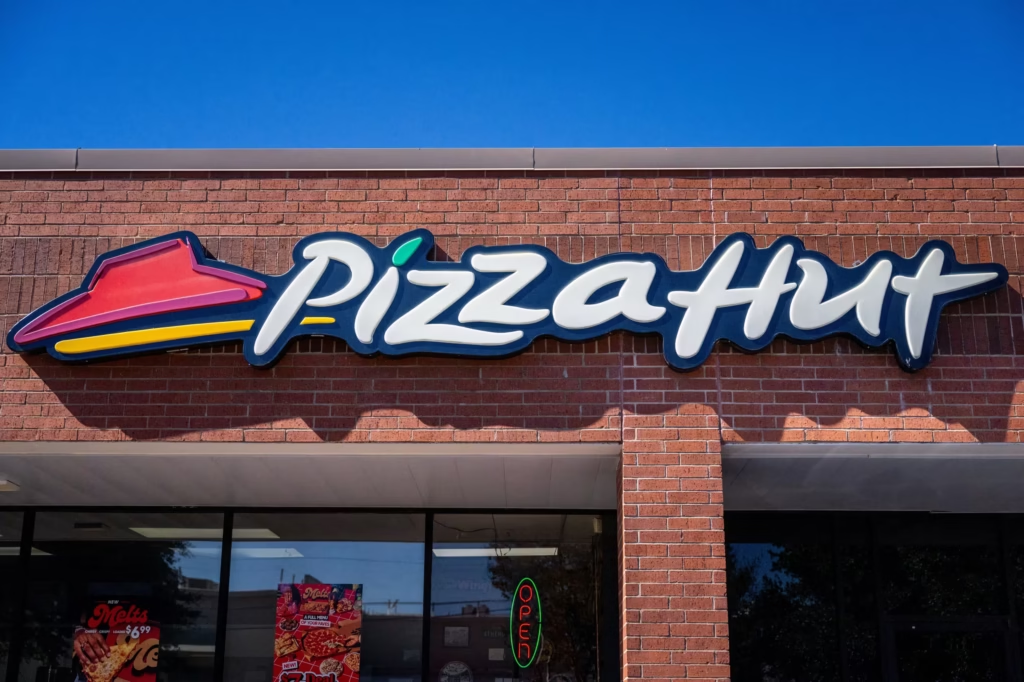
1.2 Disruptive Paradigms and New Value Levers
The impact of AI extends far beyond cost cutting. It creates entirely new revenue streams and enhances operational agility. Three main areas illustrate this shift:
- Personalized Customer Experience:
As exemplified by Yum Brands, AI-driven personalization transforms the traditional, one-size-fits-all marketing approach. Advanced algorithms process customer data—from purchase histories to behavioral insights—to tailor interactions in real time. This strategy not only improves conversion rates but also deepens brand loyalty.
Yum Brands AI - Operational Agility and Process Optimization:
Telstra’s joint venture with Accenture is a compelling case in point. By reconfiguring its organizational structure and consolidating its vendor relationships, Telstra is accelerating the deployment of AI-driven solutions across its operations. This integration reduces friction in workflows, drives faster decision-making, and ultimately creates a leaner, more responsive enterprise.
Telstra & Accenture - Innovation in Product and Service Delivery:
Companies like AMD under Lisa Su are integrating AI end-to-end across their product lines. AMD’s aggressive M&A strategy—including the acquisition of Xilinx, Pensando, and Silo AI—illustrates its commitment to growth strategy embedding AI in every aspect of its operations, from chip design to customer-facing applications. This approach creates a “virtuous cycle” where improved AI capabilities drive better products, which in turn generate more data to refine the AI further.
Lisa Su on AMD’s AI Strategy
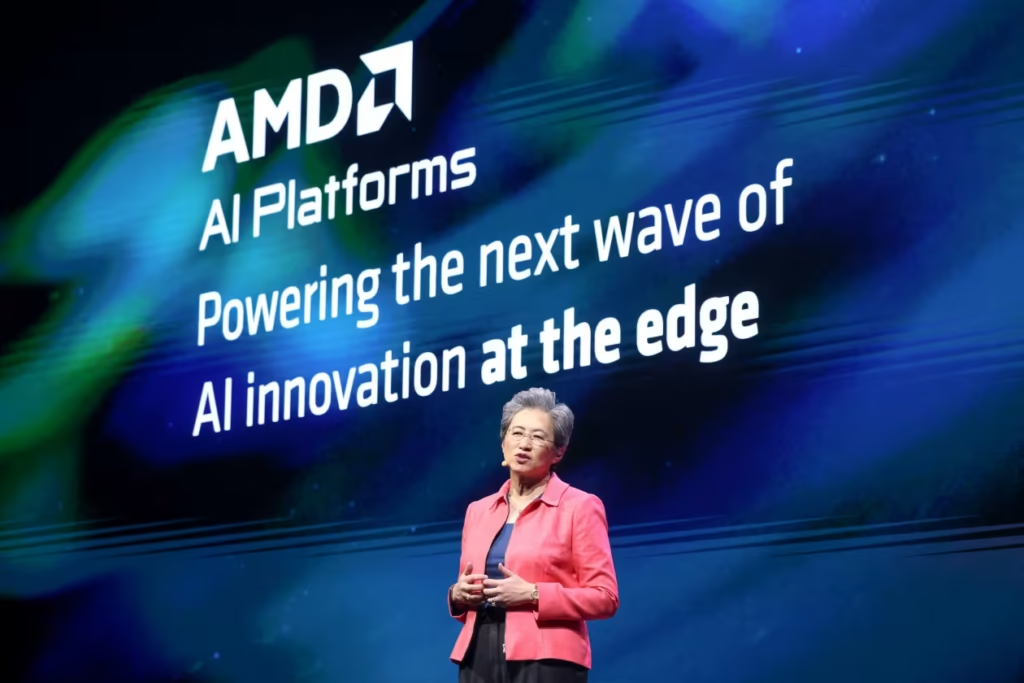
These disruptive paradigms demand a rethinking of conventional growth strategies. Enterprises must now evaluate how AI reshapes value creation and how their competitive advantage can be sustained by not only adopting new technologies but by fundamentally reimagining business models.
2. A Nuanced, Multi-Dimensional AI Growth Framework
Moving beyond the standard five-phase model, our framework recognizes that true AI integration requires addressing multiple interdependent dimensions: strategic vision, data maturity, operational re-engineering, ecosystem integration, and continuous innovation. Each of these dimensions interacts dynamically to produce a sustainable, AI driven growth strategy engine.
2.1 Strategic Vision Coupled with Data Maturity
Vision Beyond Pilots:
It is imperative that senior leadership formulates an AI strategy that transcends isolated pilots. Rather than treating AI as an add-on project, companies must integrate AI into their core vision—embedding it into every decision-making process and product line. This involves rigorous scenario planning and foresight in growth strategy. CEOs need to ask not only, “What markets can we capture?” but also, “How can AI reshape our value proposition?” Executives must balance short-term wins with long-term transformation.
Data as the Differentiator:
High-quality, unified data is the lifeblood of effective AI. According to KPMG’s global head of AI, organizations will increasingly be differentiated by the quality and uniqueness of their data sets. Building robust data pipelines, ensuring data integrity, and maintaining stringent governance practices are essential. Companies that can seamlessly integrate data from disparate sources are better positioned to train AI models that yield actionable, accurate insights in their growth strategy.
Data Governance & KPMG Insights
2.2 Integrated Digital Architecture and Talent Ecosystem
End-to-End Integration:
For AI to deliver on its promise, it must be embedded within the enterprise’s entire digital architecture. This means re-engineering existing systems to support real-time data flows and integrating AI with core enterprise applications like ERP, CRM, and supply chain management systems. Cloud-native AI solutions offer the scalability and flexibility required for this integration, allowing companies to adapt rapidly as data volumes and model complexities increase. This architectural overhaul is not just about technology—it’s about creating an agile, responsive operating system for the enterprise.
Talent and Partnership Strategies:
The shortage of AI expertise remains a formidable challenge. Successful companies adopt a hybrid approach: they upskill existing employees while strategically acquiring external talent through M&A. AMD’s acquisitions of Xilinx, Pensando, and Silo AI are prime examples of how targeted talent and IP acquisition can rapidly elevate a company’s AI capabilities. Moreover, fostering strategic partnerships with leading technology providers can mitigate talent gaps and accelerate innovation cycles.
AMD’s AI M&A Strategy
2.3 Operational Re-Engineering for AI Agility
Agile Process Reconfiguration:
Traditional workflows are often rigid, inhibiting rapid experimentation and iterative improvement. To unlock AI’s full potential in growth strategy, organizations must redesign their processes to be agile. This includes establishing AI “sandboxes” that allow for continuous testing and integration of new models without disrupting ongoing operations. Agile methodologies enable teams to iterate quickly, adapt to emerging insights, and scale successful pilots into enterprise-wide deployments.
Redefining Performance Metrics:
Conventional KPIs might not capture the multifaceted benefits of AI. Organizations need to develop new metrics that evaluate AI-specific outputs—such as model accuracy, prediction speed, and the incremental value delivered by AI insights. These metrics should be linked directly to strategic objectives, ensuring that AI initiatives drive tangible improvements in business performance.
Case in Point – Predictive Maintenance:
Predictive maintenance systems in manufacturing illustrate these principles. By analyzing sensor data from equipment, AI models can predict failures before they occur, allowing for timely interventions. This not only reduces downtime but also extends the operational lifespan of critical machinery. Companies that have adopted such systems report cost savings of 20–30% and significant improvements in operational reliability.
2.4 Ecosystem Reconfiguration and Network Effects
Partnering for Scale:
No company operates in a vacuum. The integration of AI into an enterprise’s growth strategy is greatly enhanced by building a robust ecosystem of partners. Telstra’s joint venture with Accenture is an instructive example: by combining internal capabilities with Accenture’s global expertise, Telstra has accelerated its AI deployment across operations. Such partnerships create network effects, where shared expertise and resources multiply the value delivered by each player in the ecosystem.
Telstra & Accenture Partnership
Co-Innovation Platforms:
Establishing platforms where internal teams, external partners, academia, and even customers can collaborate is critical. These co-innovation hubs foster a culture of experimentation and rapid prototyping, leading to breakthrough innovations that might otherwise remain unrealized. For instance, as part their growth strategy, several leading companies are exploring the concept of “AI factories” (AI Factory – Wikipedia)—integrated systems that continuously learn from data, refine algorithms, and generate operational insights in a virtuous cycle.
2.5 Continuous Learning and Future-Proofing
Feedback Loops and Adaptive Governance:
The pace of AI evolution demands that enterprises establish robust feedback mechanisms. Continuous monitoring of AI performance, combined with adaptive governance frameworks, ensures that models remain accurate and aligned with strategic goals. As new data streams emerge and market conditions change, AI systems must be recalibrated to prevent model drift and maintain efficacy. Leaders must also institute ethical guidelines and oversight mechanisms to address biases and ensure transparency.
AI Governance Insights – Wired
Investing in Next-Gen AI Capabilities:
Future-proofing an enterprise means looking beyond current AI applications and investing in next-generation technologies such as multi-agent systems and advanced generative AI. These emerging technologies promise to unlock even greater value by enabling complex reasoning, real-time decision support, and fully autonomous operations. Progressive companies are already earmarking budgets for R&D initiatives that explore these frontier technologies, ensuring they remain at the cutting edge as the AI landscape evolves.
3. Deep-Dive Discussion: Strategic Implications and Tactical Considerations
3.1 Balancing Speed with Prudence
Rapid AI deployment is critical, yet premature integration without proper safeguards can lead to pitfalls. Business leaders must balance the need for speed with a methodical, risk-aware approach. For example, the AP article on AI’s rapid integration warns that while nearly half of Fortune 1000 companies have embedded AI in their workflows, many still face issues like data “hallucinations” in customer-facing applications. Implementing rigorous “maturity tests” ensures that AI systems are thoroughly vetted before scaling. Leaders should institute trial phases and comprehensive validation frameworks to gauge AI readiness, thereby protecting their operations from unforeseen consequences.
AP on AI Maturity
3.2 Organizational Transformation and Change Management
The transition to an AI-centric business model is as much about people as it is about technology. Research from Deloitte and McKinsey underscores that while employees are ready for AI, significant skills gaps exist. Leaders must therefore create a culture that embraces change through continuous learning and agile decision-making. This involves:
- Redesigning Job Roles: Rather than viewing AI as a threat, companies should reconfigure roles to leverage AI as an augmentative tool. For instance, customer service representatives can be supported by AI chatbots that handle routine queries, allowing humans to focus on complex, empathetic interactions.
- Upskilling and Reskilling: Implement formal training programs to bridge the AI skills gap. McKinsey’s report on “Superagency in the Workplace” highlights the importance of investing in training, as employees often underestimate their AI capabilities.
Superagency in the Workplace – McKinsey - Change Management Strategies: Engage employees early in the AI integration process. Transparency, frequent communication, and incentive schemes can ease apprehension and foster a sense of shared purpose. CEOs must lead by example, actively participating in training and demonstrating the value of AI in everyday operations.
3.3 Navigating Regulatory and Ethical Challenges
With AI’s deep penetration into business operations, ethical considerations and regulatory compliance become non-negotiable. Data privacy, bias, and accountability are pressing issues that require:
- Robust Data Governance: Establish clear protocols for data collection, storage, and usage. This ensures that AI models are trained on unbiased, high-quality data and that sensitive information is protected against breaches.
- Ethical AI Frameworks: Create ethics boards and implement transparent AI models where decision pathways are explainable. KPMG’s initiatives in AI strategy emphasize the importance of integrating ethics into AI strategy to prevent misuse and maintain customer trust.
KPMG AI Strategy - Regulatory Readiness: Stay ahead of evolving regulations. With different markets enforcing varied data protection laws, companies must build flexible systems capable of meeting global compliance standards. As PwC’s Chief AI Officer Dan Priest advises, organizations that scale AI responsibly—much like early internet adopters—will secure lasting competitive advantage.
AP on AI in 2025
3.4 Competitive Dynamics and Market Repositioning
The integration of AI is not just an operational upgrade—it fundamentally shifts market dynamics. Enterprises that successfully integrate AI in growth strategy can achieve:
- Sustainable Competitive Advantage: Companies that harness AI to deliver personalized customer experiences, optimize supply chains, and drive innovation can differentiate themselves in crowded markets. For instance, Alibaba’s aggressive AI investments have driven an 8% revenue surge and positioned the firm at the forefront of China’s AI race.
Alibaba Revenue Surge on AI - Agility and Resilience: AI-enabled predictive analytics allow companies to anticipate market shifts and adjust strategies in real time. This responsiveness is critical in today’s volatile business landscape. Dell’s remarkable revenue growth in its Infrastructure Solutions Group is partly attributed to its rapid AI infrastructure rollouts, enabling it to capture new market opportunities.
Dell’s AI Transformation - Ecosystem Synergies: By forming strategic alliances—like those between Telstra and Accenture or AMD with its tech partners—companies can create synergies that amplify innovation and deliver integrated solutions across the value chain. Such ecosystems drive value far beyond what isolated AI projects could achieve.
3.5 The Role of Leadership in the AI Transformation Journey
Despite high employee readiness, our research reveals a significant leadership gap. Only a small fraction of C-suite executives describe their AI initiatives as “mature.” Leaders must:
- Adopt a Bold, Forward-Looking Mindset: Recognize that AI is a catalyst for transformation, not a mere tool for automation. Visionary leaders must articulate an AI strategy that encompasses long-term growth, ethical considerations, and workforce transformation.
- Set Clear, Measurable Goals: Define what success looks like for AI initiatives. This involves setting up KPIs that track not just efficiency gains, but also the broader impact on business outcomes, customer experience, and market position.
- Invest in Human Capital: Provide ongoing training, encourage cross-functional collaboration, and create an environment where failure is seen as a learning opportunity. As Lisa Su of AMD emphasizes, the key to success lies in combining technological prowess with strong, visionary leadership.
Lisa Su on AMD’s AI Strategy
4. Case-Informed Insights Across Industries
To truly understand the transformative potential of AI, let’s explore detailed case studies from various industries that illustrate the application of our framework.
4.1 Healthcare: Revolutionizing Patient Care and Diagnostics
Case Study – IBM Watson Health & AlphaFold:
IBM Watson Health and DeepMind’s AlphaFold are pioneers in applying AI to healthcare. IBM Watson Health uses AI to sift through enormous volumes of clinical data, research papers, and medical imaging to support diagnostics and personalized treatment. DeepMind’s AlphaFold has revolutionized our understanding of protein folding—a breakthrough that accelerates drug discovery and precision medicine. These systems not only enhance diagnostic accuracy but also reduce the time required to develop life-saving treatments.
Key Strategic Lessons:
- Data Quality is Paramount: The success of these AI systems hinges on having access to clean, comprehensive, and up-to-date clinical data.
- Cross-Disciplinary Collaboration: Healthcare AI requires close collaboration between medical professionals, data scientists, and regulatory experts to ensure that technology is both effective and compliant with stringent healthcare standards.
- Predictive Analytics for Preventative Care: By forecasting potential health risks, AI can facilitate early intervention, significantly improving patient outcomes and reducing long-term costs.
4.2 Manufacturing and Industrial Sectors: Enhancing Efficiency and Reducing Downtime
Case Study – Predictive Maintenance in Manufacturing:
Leading manufacturing companies have adopted AI-powered predictive maintenance systems to monitor equipment performance and forecast potential failures. For instance, Siemens has implemented advanced sensors and machine learning models to predict machine breakdowns before they occur, thereby reducing unplanned downtime by up to 30%. These AI-driven systems not only save costs but also extend the operational lifespan of critical machinery.
Key Strategic Lessons:
- Real-Time Data Integration: Successful predictive maintenance relies on the real-time collection and analysis of sensor data, which requires robust data pipelines and cloud-based storage solutions.
- Operational Agility: By automating maintenance scheduling, companies can allocate resources more efficiently, ensuring smoother production flows and better supply chain performance.
- Scalability: As manufacturing environments vary widely, scalable AI systems that can adapt to different equipment and conditions are essential for consistent performance across global operations.
4.3 Retail and E-Commerce: Driving Personalization and Operational Excellence
Case Study – Yum Brands’ Byte by Yum!:
Yum Brands has integrated AI across its digital ecosystem through its Byte by Yum! platform. This comprehensive solution enhances mobile ordering, optimizes kitchen operations, and delivers personalized marketing. By analyzing customer behavior and purchase history, AI algorithms tailor promotions and product recommendations, resulting in double-digit improvements in revenue per email and increased conversion rates. The integration of AI not only streamlines operations but also creates a deeper, data-driven connection with consumers.
Key Strategic Lessons:
- Personalization at Scale: AI empowers retailers to move from generic to hyper-personalized marketing, significantly boosting customer engagement and sales.
- Operational Efficiency: Automating order processing and kitchen management minimizes human error and improves delivery times, reinforcing the brand’s competitive position.
- Data-Driven Insights: The ability to quickly process vast amounts of consumer data allows retailers to adapt rapidly to market trends, ensuring that product offerings remain relevant and enticing.
4.4 Financial Services: Strengthening Risk Management and Enhancing Customer Trust
Case Study – AI in Fraud Detection and Risk Management:
Financial institutions like JPMorgan Chase and Mastercard are at the forefront of using AI for fraud detection and risk management. By analyzing transactional data in real time, AI systems can flag unusual patterns indicative of fraudulent activities. These predictive models have helped reduce false declines and improve the accuracy of risk assessments, thereby protecting customer assets and enhancing overall trust.
Key Strategic Lessons:
- Integration of Predictive Analytics: The deployment of AI in risk management enables real-time threat detection, allowing companies to take swift action before minor issues escalate.
- Balancing Automation with Human Oversight: While AI can handle large-scale data analysis efficiently, human judgment remains crucial in verifying and acting on AI-driven insights.
- Regulatory Compliance: Robust AI systems that incorporate explainability and transparency are essential for meeting stringent regulatory requirements and ensuring that risk management practices are both ethical and effective.
WSJ on AI in Finance
4.5 Telecommunications and IT: Building Future-Proof Digital Infrastructures
Case Study – Telstra’s Joint Venture with Accenture:
Telstra’s strategic partnership with Accenture is a prime example of how companies are reconfiguring their operational structures to accelerate AI adoption. By creating a joint venture, Telstra is able to centralize its AI initiatives, streamline vendor relationships, and rapidly scale its digital transformation efforts. This partnership not only expedites the rollout of AI solutions across the organization but also fosters cross-functional collaboration that drives innovation and operational agility.
Key Strategic Lessons:
- Strategic Alliances: Forming partnerships with technology leaders can overcome internal resource constraints and accelerate AI integration.
- Organizational Re-Engineering: Restructuring internal teams and consolidating vendor relationships simplifies complex digital ecosystems, paving the way for scalable AI adoption.
- Ecosystem Integration: Leveraging external expertise while aligning internal capabilities is critical to building a comprehensive AI-driven infrastructure that supports long-term growth.
Telstra & Accenture
4.6 Semiconductor and High-Performance Computing: Driving the AI Hardware Revolution
Case Study – AMD’s End-to-End AI Integration:
Under the visionary leadership of CEO Lisa Su, AMD has transformed from a struggling chipmaker into a powerhouse in high-performance computing. Through strategic acquisitions—including Xilinx, Pensando, and Silo AI—AMD has integrated AI capabilities across its entire product portfolio. Its MI300 and MI300X AI chips, which rival industry giants like Nvidia’s H100, represent the pinnacle of AI-optimized hardware. This holistic approach enables AMD to offer end-to-end solutions that combine advanced processing capabilities with integrated AI functionality.
Key Strategic Lessons:
- Holistic Integration: The synergy between hardware and software is crucial for creating high-performance, AI-powered products.
- Strategic M&A: Acquiring specialized talent and technology through M&A accelerates innovation and enhances competitive positioning.
- Global Partnerships: Collaborating with key players like Microsoft, Meta, and Oracle not only broadens market reach but also validates the strategic vision of integrating AI end-to-end.
AMD AI Strategy – Time
4.7 ESG and Sustainability: Leveraging AI for Responsible Growth
Case Study – Leveraging AI for ESG Goals:
Fortune 500 companies are increasingly integrating AI into their Environmental, Social, and Governance (ESG) strategies to drive sustainable business practices. Recent research has demonstrated that AI can help organizations reduce energy consumption, lower carbon emissions, and enhance transparency in ESG reporting. By using AI-powered analytics, companies can quickly process environmental data to identify inefficiencies, predict future resource needs, and implement cost-effective, sustainable solutions. This integration not only bolsters compliance with global ESG standards but also improves corporate reputation and investor confidence.
ResearchGate – Leveraging AI for ESG Goals
Key Strategic Lessons:
- Data-Driven Sustainability: High-quality, real-time data is critical to monitoring ESG initiatives and making informed adjustments that reduce environmental impact.
- Integrative Reporting: AI can streamline ESG reporting, ensuring that sustainability metrics are accurate, timely, and actionable.
- Long-Term Value Creation: Integrating ESG considerations into the AI strategy not only fulfills regulatory requirements but also unlocks new market opportunities by aligning with the values of conscious consumers.
5. Strategic Implications and Key Considerations
As enterprises strive to harness AI for sustainable growth, several strategic implications warrant careful consideration:
5.1 Data and Infrastructure as the Bedrock of AI Strategy
The success of any AI initiative depends on the quality, accessibility, and governance of data. Enterprises must invest in robust data architectures that integrate data from multiple sources, cleanse and standardize it, and make it readily available for AI models. This includes:
- Building Unified Data Pipelines: Eliminating silos is paramount. A centralized data infrastructure allows for continuous model training and improved predictive accuracy.
- Ensuring Data Security and Privacy: As data breaches become more frequent, companies must adhere to stringent data protection protocols and regulatory standards to maintain trust and avoid legal repercussions.
- Leveraging Cloud Solutions: Cloud-native platforms provide scalability and flexibility, essential for handling the computational demands of advanced AI models.
5.2 Talent, Culture, and Change Management
Bridging the AI skills gap is one of the biggest challenges facing enterprises today. While cutting-edge technology is crucial, the human element remains irreplaceable:
- Upskilling and Reskilling Programs: Investing in continuous learning initiatives ensures that employees are equipped to work alongside AI, thereby enhancing overall productivity.
- Fostering a Culture of Innovation: Organizational transformation is not just about technology—it’s about shifting the culture to embrace change, experimentation, and cross-functional collaboration.
- Redefining Leadership Roles: Leaders must transition from traditional supervisory models to roles that focus on strategic oversight, human-AI collaboration, and continuous improvement.
McKinsey Superagency Report
5.3 Governance, Ethics, and Regulatory Compliance
With AI’s deep penetration into business operations, ethical considerations and regulatory compliance become non-negotiable. Companies must:
- Establish Robust Data Governance: Clear protocols for data collection, storage, and usage are essential.
- Develop Ethical AI Frameworks: Transparency, accountability, and bias detection must be integral to all AI systems.
KPMG AI Strategy - Maintain Regulatory Readiness: Build flexible systems capable of adapting to global data protection laws.
AP on AI Regulations
5.4 Innovation and Competitive Advantage
Integrating AI is not just an upgrade—it redefines market dynamics:
- Unlocking New Revenue Streams: AI-powered personalization, predictive analytics, and automation open new markets and business models.
- Enhancing Customer Experience: Customized interactions lead to higher satisfaction and loyalty.
- Driving Operational Agility: Rapid, data-driven adjustments in strategy give companies a decisive edge in volatile markets.
- Ecosystem Synergies: Strategic partnerships (e.g., Telstra & Accenture, AMD’s alliances) multiply the benefits of AI integration.
WSJ on AI Infrastructure Investments
5.5 Ecosystem and Partnership Integration
Building a robust AI ecosystem is essential:
- Form Strategic Alliances: Collaborations with tech leaders, academic institutions, and even competitors can accelerate AI integration.
- Create Co-Innovation Platforms: Collaborative hubs foster breakthrough innovations through shared expertise.
6. Long-Term Vision: AI-Native Organizations and the Future of Work
6.1 The Evolution of AI Maturity
Today, many companies are still in the pilot phase of AI integration in their growth strategy; only about 1% of Fortune 1000 companies consider their AI deployments fully mature. Achieving maturity involves:
- Scaling Successful Pilots: Systematically roll out proven AI initiatives across the enterprise.
- Building Resilient AI Systems: Ensure systems are reliable, transparent, and adaptive.
- Institutionalizing Continuous Improvement: Ongoing investment in R&D and regular recalibration are critical.
6.2 The Impact on Organizational Structure
AI integration is expected to fundamentally shift organizational structures. We may see:
- Dynamic Team Formation: Fluid, project-based teams formed around specific objectives.
- Evolving Middle Management: Managers transitioning to roles focused on human-AI coordination.
- Empowered Frontline Employees: Enhanced decision-making capabilities at all levels.
Wired on AI and Organizational Strategy
6.3 The Promise of AI Superagency
“Superagency” envisions a future where AI amplifies human capabilities:
- Enhanced Creativity: Automation of routine tasks frees up time for innovation.
- Democratization of Expertise: AI bridges knowledge gaps across the organization.
- Transformational Leadership: Leaders who blend human and AI capabilities will drive breakthrough innovations.
Wired on AI Superagency
6.4 Economic and Societal Implications
The economic potential of AI is vast, with studies by McKinsey estimating trillions in productivity gains globally. However, this transformation also brings societal responsibilities:
- Workforce Transformation: Upskilling and reskilling programs must address job evolution.
- Ethical AI Practices: Transparent, responsible AI deployment is critical.
- Global Competitiveness: Companies that master AI integration will define future markets.
AP on AI’s Economic Impact
7. Practical Steps for Implementing AI Driven Growth Strategy
For executives ready to take the leap, here is a detailed roadmap for embedding AI into your enterprise strategy:
7.1 Assessment and Planning
- Conduct a Comprehensive AI Audit: Evaluate your data infrastructure, talent, and digital capabilities.
- Set Clear Objectives: Define specific, measurable goals for AI initiatives.
- Map Use Cases to Business Outcomes: Prioritize high-ROI projects.
- Engage Stakeholders Early: Involve IT, operations, finance, and marketing leaders in planning.
7.2 Building the Foundation
- Invest in Data Infrastructure: Upgrade data pipelines and establish robust governance.
- Develop Talent and Partnerships: Upskill employees and consider strategic acquisitions (e.g., AMD’s Silo AI acquisition).
AMD AI Strategy – Time - Establish an AI Center of Excellence (CoE): Centralize AI innovation and standardize practices.
- Pilot and Iterate: Start with small projects, learn quickly, then scale.
7.3 Scaling and Integration
- Embed AI into Core Processes: Automate workflows and integrate AI with ERP, CRM, and supply chain systems.
- Monitor Performance and Optimize Continuously: Use dashboards and KPIs to track progress.
- Foster a Culture of Innovation: Incentivize experimentation and reward successful AI initiatives.
- Leverage Ecosystem Partnerships: Expand capabilities through strategic alliances.
7.4 Ensuring Long-Term Sustainability
- Create Adaptive Governance Structures: Set up ethics boards and oversight committees.
- Focus on Continuous Learning: Implement ongoing training and foster a culture of lifelong learning.
- Invest in Future Technologies: Allocate resources for R&D into next-gen AI.
- Align AI Strategy with ESG Goals: Integrate AI with sustainability initiatives to enhance corporate reputation.
ResearchGate on AI for ESG Goals
8. Future Trends and the Road Ahead
Looking forward, several trends are set to define the AI-powered enterprises of tomorrow:
8.1 Enhanced Predictive Analytics and Real-Time Decision-Making
Next-gen AI models will offer unprecedented predictive capabilities, allowing companies to make proactive decisions in real time. This transformation will enable organizations to adapt quickly to market shifts and consumer trends.
8.2 Deep Integration into Organizational DNA
The most successful companies will be those that embed AI throughout their operations—not just as a tool, but as an integral component of their strategy and culture. This deep integration will drive innovation, streamline processes, and foster a more agile organization.
8.3 The Rise of AI Superagency
The concept of superagency—where AI amplifies human productivity and creativity—will be a major differentiator. Companies that empower their workforce with advanced AI tools will see exponential benefits in innovation and efficiency.
Wired on AI Superagency
8.4 Economic and Societal Impacts
The productivity gains from AI could add trillions to the global economy. However, organizations must address workforce transformation, ethical AI practices, and regulatory challenges to fully realize these benefits.
AP on AI’s Economic Impact
8.5 Industry-Specific Innovations
Different sectors will experience unique transformations:
- Healthcare: Enhanced diagnostics, virtual clinicians, and personalized treatments.
- Manufacturing: Digital twins, IoT integration, and predictive maintenance.
- Retail: Hyper-personalization, dynamic inventory management, and customer journey enhancements.
- Financial Services: Advanced fraud detection, risk management, and AI-driven customer support.
- Telecommunications: Optimized networks and infrastructure management.
- Semiconductors: Breakthroughs in AI-enabled chip design and production.
- Sustainability: AI-integrated ESG strategies to drive environmental responsibility.
AP on Alibaba’s AI Growth
9. The Imperative for Visionary Leadership in an AI Driven Growth Strategy
Despite the vast potential of AI, the transformation journey is fraught with challenges. While employees are ready and enthusiastic about AI adoption, our surveys reveal that only a small percentage of senior executives consider their AI initiatives fully mature. This leadership gap underscores the need for bold, visionary leadership:
- Visionary Leadership:
Leaders must set ambitious AI targets and articulate a strategy that integrates AI into the core of the business. As exemplified by AMD’s transformative journey under CEO Lisa Su, success hinges on leaders who can combine technological prowess with strategic vision.
Lisa Su on AMD’s AI Strategy - Creating a Culture of Innovation:
Continuous learning, upskilling, and cross-functional collaboration are essential. Organizations must redesign job roles to leverage AI as an augmentative tool rather than a replacement, ensuring that human judgment and creativity remain central. - Change Management and Adaptability:
Leaders must proactively address resistance, communicate transparently, and establish mechanisms for continuous feedback. The goal is to create an environment where AI adoption is seen as a pathway to growth and innovation, not as a threat to existing roles. - Ethical Leadership:
Integrating AI responsibly is critical. This involves establishing robust governance frameworks, ensuring data privacy, and maintaining transparency in AI decision-making processes. Ethical AI is not just a regulatory necessity—it builds long-term trust with customers and employees.
10. Conclusion: Embarking on the AI Transformation Journey
The convergence of advanced AI technologies, robust data infrastructures, and visionary leadership is setting the stage for a transformative shift in enterprise growth strategies. Companies that recognize AI as a fundamental driver of change—and not just a tool for automation—will be well-positioned to capture significant value in the coming years.
This comprehensive framework shows that successful AI integration requires more than technology adoption. It demands a rethinking of organizational structures, a commitment to continuous learning, and the establishment of ethical and adaptive governance practices. By addressing these critical dimensions, enterprises can transform AI from a set of isolated projects into a cohesive, strategic asset that permeates every facet of the business.
Fortune 500 companies and global industry leaders are already reaping the benefits of AI-driven transformation. From enhancing predictive analytics and operational efficiency to creating personalized customer experiences and unlocking new revenue streams, the potential is enormous. Yet the true challenge lies in scaling these innovations sustainably while ensuring that the human element remains central to decision-making and value creation.
As you, the C-level executive, consider your organization’s future, ask yourself: How will you not only adopt AI but truly become an AI-native enterprise? The answer lies in embracing a bold vision, investing in data and talent, re-engineering processes, and fostering a culture of continuous innovation. The journey is complex, but the rewards—a competitive edge in an increasingly digital world—are too significant to ignore.
Leaders must now step up, not just to automate but to reinvent. The time to act is now. Those who can seamlessly blend human ingenuity with AI capabilities will define the next wave of global enterprise growth, unlocking efficiencies, sparking innovation, and transforming industries for decades to come.
In depth analysis, strategic insights and proposed framework in this report are are powered by Nexstrat.ai. To explore how it can enhance your growth strategy development, schedule a demo at nexstrat.ai/book-a-demo.
About NexStrat AI:
NexStrat AI is at the forefront of AI and business strategy innovation. As the ultimate strategy and transformation AI co-pilot and platform, we help leaders and strategists craft winning strategies and make effective decisions with speed and confidence.
Contact Us:
Have questions or want to learn more? Contact us at [email protected]
Follow Us:
Join our community of forward-thinking business leaders on LinkedIn.